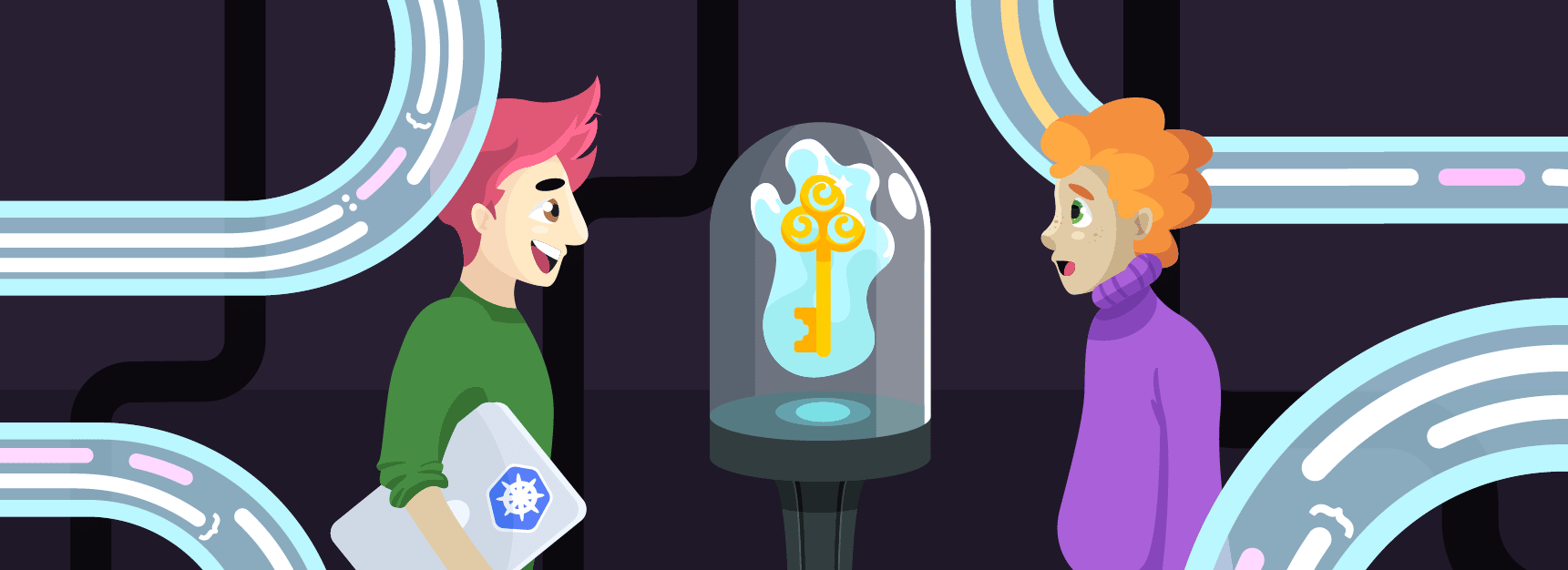
Is IT Suffocating Your Organization? Here’s How to Get Your Contextual Data Pipelines Right
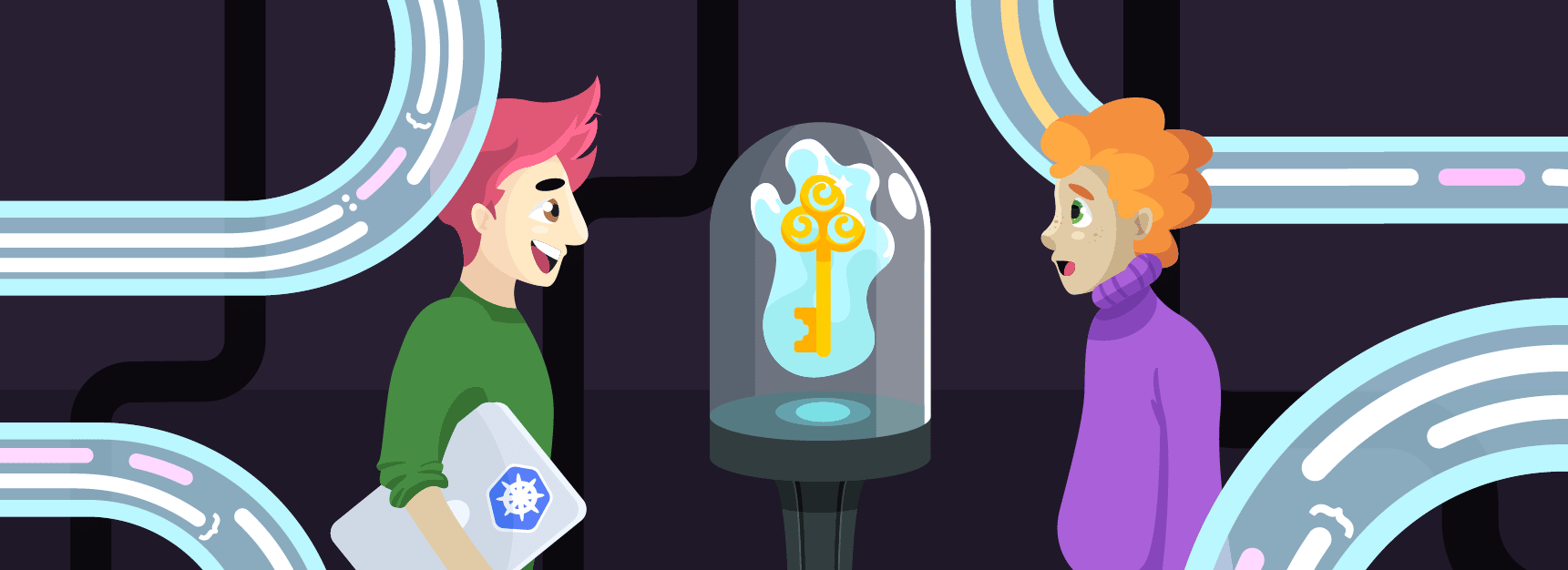
In a modern organization, the dependency on constant data flow doesn’t skip a single role — already encompassing every function in R&D, Sales, Marketing, BI, and Product. Essentially every position is going through a fusion process with data-science.
“Data is the new oil.” “Everyone needs data.” You’ve probably run into these and similar expressions more than once. The reason you hear them so often is that they are true. In fact, those sayings are becoming truer by the minute.
Software is naturally data-driven but AI is naturally data-hungry. As both fields are currently experiencing exponential growth, more and more jobs connect with and depend on software, and as a result, rely on data access. We can already see the effects rippling across the job market. As Ollie Sexton of Robert Walters PLC, a global recruitment agency points out:
“As businesses become ever more reliant on AI, there is an increasing amount of pressure on the processes of data capture and integration… Our job force cannot afford to not get to grips with data and digitalization.”
Data is not the new oil, It’s the new oxygen
As the demand for data explodes, the stress on our data pipelines increases. Not only do we need to generate more data faster but also to generate quality, contextual data – i.e. the right data points, with the right context, at the right time.
Generally speaking, the problem is being unable to see the forest for the trees. If we try to record all data all the time, we will end up drowning in a data deluge. Thus, the challenge becomes not about data, but about knowledge and understanding. In other words, it’s all about contextual data. To drive the point home, let’s look at three example test cases and consider how different fields are impacted by the growth of data and software.
Cyber Security
Software is everywhere and so, every corner may serve as a potential attack surface. Nonetheless, trying to record all communications, interactions, and actions would quickly leave us distracted or blind to real attacks when they happen. Organizations can respond in a timely fashion and maintain their security posture only in three specific manners: by mapping the attack surface with the right context, e.g. weakest links and key access points; setting the right alerts and traps, and; by zooming in to generate more comprehensive data on attacks as they happen.
Debugging
Software is continually becoming more complex and interconnected. Replicating it to simulate debugging/development scenarios has become costly and ineffective. Trying to monitor all software actions all the time in hopes of catching the root cause of a bug or an issue comes with even greater compute and storage costs, not to mention the significant maintenance costs. Worst of all, the data deluge will blind developers, as they spend more and more time sifting through growing piles of logging data, looking for needles in haystacks. So how can developers obtain the key data points they need to identify the root cause of issues, fix, and improve the software? Only by being laser-focused on contextual, real behaviors or incidents and diving deep into the software as these incidents happen in real-time.
User analytics
More software means more user interactions. More interactions mean more behavior patterns to identify, and as users and software evolve — so do the patterns. Trying to constantly record all the interactions will result in too much time wasted on processing irrelevant patterns which would become obsolete by the moment they are identified. How can product designers and BI scientists stay ahead (or even alongside) of the curve? Only by focusing on the important contextual interactions, and by identifying them and their core patterns quickly as they appear and change.
Ultimately, for modern organizations, there is no avoiding a significant infrastructure effort to pipeline contextual data wherever and whenever it is needed. Without that contextual data, your organization’s departments will wither, fading into gross inefficiency and irrelevance — becoming legacy and obsolete. As Tsvi Gal, CTO of infrastructure at Morgan Stanley put it:
“We [may be] in banking, but we live and die on information…. Data analytics is the oxygen of Wall Street.”
Context? Go straight to the source
Context is driven by perspective. For instance, the BI team and the R&D team will require different contexts from the same data sources. One of the biggest challenges with getting contextual data is that data processing and aggregation are inherently subjective and may cause the needed context to be lost. For example, “these parts are important, and these are not”; “these data-points can be combined and saved as one, and these must remain unique.”
There’s only one way to guarantee the right context with the right data sets. Consumers of the data must be allowed to define their required context and apply it to the collection of the data right at the source. This is no easy feat, as the source is not only defined in space and content but also (and maybe more critically) in time.
Getting data at the source is hard
Every data pipeline contains two parts. A source — the way we extract or collect the data, and a destination — the way we bring the data where it needs to be processed, aggregated, or consumed. Automation has greatly improved the destination part, with impressive tools like Tableau, Splunk, ELK stack, or even the cloud-native EFK stack powered by FluentD. Yet sourcing data still remains a largely manual engineering process. Aside from predefined envelope analytics like mouse clicks, network traffic, and predefined user patterns, it still requires extensive design, engineering, and implementation cycles. As a result, the key to data sourcing still remains withheld from a large part of the organization.
Who currently has the key?
With the required engineering, traditionally R&D and IT hold the key to collecting and pipelining data to the various consumers within the organization. Although this arrangement appears natural at first glance, it quickly translates into a significant bottleneck problem.
As the demand for contextual data grows across the organization, pressure on R&D and IT increases and gradually becomes a significant percentage of their work. Context-switching between development work and addressing the arising data requests results in slow response rates to data needs, frustrated data consumers across all departments, and constant friction on all R&D efforts.
Who should hold the key?
If your answer to the question above includes R&D, IT, analysts, or any other role, you haven’t been paying attention. The fact that the keys to data pipelines currently lie in the hands of any single department within a company is the very cause of the aforementioned bottlenecks and slower organizational processes. The systems we are building are becoming more complex and distributed. Similarly, we need to meet the complexity and distribute access to contextual data at its source.
In other words, removing bottlenecks, allowing data consumers to access the exact contextual data they need just when they need it, and enabling the various people to flourish at their jobs, requires the democratization of data sourcing and the pipelining process.
As Marcel Deer has aptly put it:
“In this environment, the old ways don’t work anymore. We have to focus on more human characteristics. This is a people and process issue. We need to make it easier for people to find the right data, build on it, and collaborate. And for that to happen, we must help people trust the data.”
Democratizing data sourcing and pipelining
The data democratization process involves recognizing the importance of contextual data and letting go of the false promise of the “let’s collect it all” approach. It requires investing in data sourcing technologies/infrastructure and building it with distributed access in mind so that it empowers positions across the organization.
This is not a far-fetched idea. After all, organizations have already gone through similar transitions in the past. Just try and imagine BI analysts working to figure out the next strategy without real-time analytics, or IT attempting to keep track of application stability without Application Performance Monitoring.
At this point in time, the bottlenecks on contextual data sourcing are already becoming painful and detrimental to the performance of most organizations. With software and data only growing with increasing speed, the pain and friction are on the cusp of turning into full deadlocks. Now is the time to give every department a key to unlocking this deadlock. It is time to liberate data and empower people.